Addressing Bias and Fairness in AI : In swiftly evolving landscape of artificial intelligence (AI) United States stands at vanguard of innovation and implementation.
As AI systems become an increasing number of integrated into numerous components of American society from healthcare and finance to criminal justice and schooling crucial challenge has emerged: presence of bias in AI and imperative to ensure fairness in those structures.
This issue has garnered sizeable interest from researchers policymakers & enterprise leaders throughout united states of america sparking countrywide communication about moral implications of AI and steps important to address these challenges.
The quest for equity and independent AI is not merely an academic exercise; it has real global consequences that have an effect on millions of Americans.
Biased AI systems can perpetuate or even exacerbate current societal inequalities leading to discriminatory effects in regions together with hiring practices mortgage approvals crook sentencing & healthcare diagnoses.
As United States continues to grapple with its complicated records of systemic racism and discrimination want to cope with bias in AI has become increasingly pressing.
This article delves into multifaceted problem of bias and equity in AI within context of United States. We will discover origins of AI bias have look at its influences throughout numerous sectors talk cutting edge efforts to deal with these challenges & dont forget destiny panorama of ethical AI improvement in America.
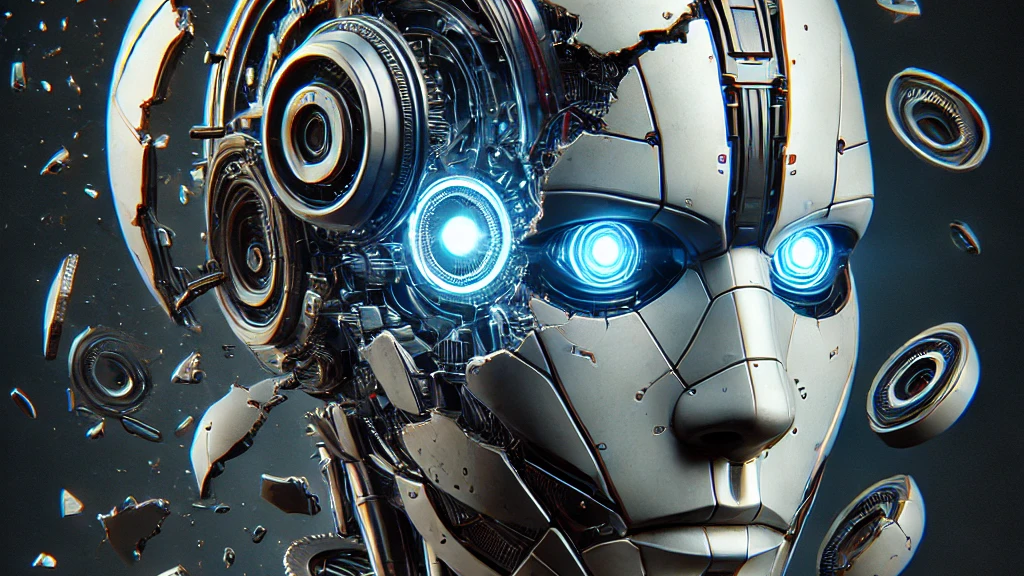
Understanding Addressing Bias and Fairness in AI inside American Context
To successfully address bias and equity in AI its miles important to first recognize what these terms suggest and how they happen within American context. AI bias refers to systematic and repeatable errors in laptop gadget that create unfair consequences which include privileging one arbitrary institution of users over others.
In United States this regularly translates to AI systems discriminating towards people based totally on race gender age socioeconomic repute or other protected characteristics.
The roots of AI bias within USA may be traced back to numerous elements. Firstly statistics used to educate AI models regularly reflects ancient biases and inequalities found in American society. For instance if an AI gadget is educated on ancient hiring statistics from organisation that has traditionally preferred male employees it can perpetuate this bias in its tips for future hires.
Secondly dearth of variety inside American tech enterprise specially in AI development groups can result in blind spots within layout and implementation of AI structures.
When creators of AI lack diverse perspectives and stories they will inadvertently build structures that fail to account for wishes and realities of underrepresented organizations.
Lastly complex interplay of societal institutional & man or woman biases in United States can take place in AI structures in diffused and often unintended ways. For example an AI machine designed to expect recidivism rates may additionally inadvertently discriminate against certain racial agencies due to ancient biases inside crook justice gadget.
Impact of Biased AI Across American Sectors
The outcomes of biased AI structures are long way accomplishing and effect diverse sectors of American society. Lets have look at few key regions where AI bias has been especially tricky:
Criminal Justice
In world of crook justice AI systems are increasingly more being used for predictive policing risk assessment in bail choices & sentencing tips. However these systems have come underneath scrutiny for potentially perpetuating racial biases. For instance 2016 ProPublica investigation found that broadly used hazard evaluation tool become much more likely to falsely flag Black defendants as destiny criminals as compared to White defendants.
Healthcare
AI in healthcare guarantees to revolutionize diagnostics remedy plans & patient care. However biased AI structures can cause disparities in health results. For instance observe published in magazine Science discovered that broadly used set of rules was much less probable to refer Black patients for additional care than White patients with same stage of contamination. This bias stemmed from set of rules usage of health prices as proxy for fitness needs inadvertently reflecting historical disparities in get entry to to care.
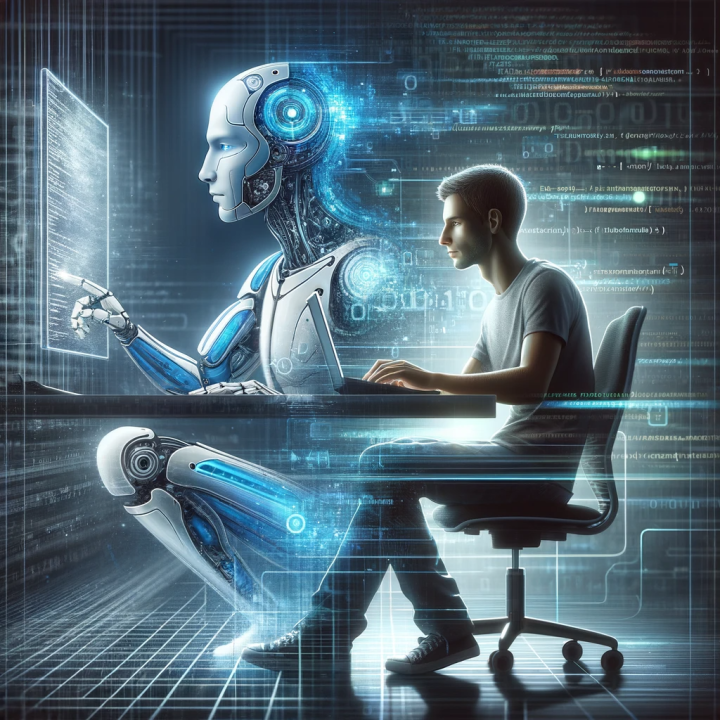
Financial Services
In financial sector AI is used for credit score scoring loan approvals & fraud detection. Biased AI systems in this area can perpetuate economic inequalities via unfairly denying credit or monetary opportunities to sure organizations. use of AI in lending selections has raised concerns approximately digital redlining wherein algorithms may also discriminate towards applicants from positive neighborhoods or demographic businesses.
Employment
AI powered hiring equipment and resume screening systems are becoming increasingly not unusual inside American job market. However those systems can perpetuate gender and racial biases if now not cautiously designed and monitored. In extremely good case Amazon had to scrap an AI recruiting tool that showed bias in opposition to women for technical jobs as it were educated on resumes submitted over 10 year period which had been predominantly from male applicants.
Education
In training AI is getting used for everything from customized getting to know platforms to college admissions decisions. Biased AI in this region can exacerbate present instructional inequalities. For instance using AI in standardized check scoring has raised worries about potential biases towards students from certain linguistic or cultural backgrounds.
Current Efforts to Address AI Bias in USA
Recognizing big challenges posed by way of biased AI numerous stakeholders inside United States are taking steps to address those issues. These efforts span academia industry government & civil society corporations.
Academic Research
American universities and research institutions are at vanguard of growing strategies to hit upon and mitigate AI bias. Researchers are working on developing more sturdy and truthful system learning algorithms developing equipment for auditing AI structures & exploring ethical implications of AI deployment.
For instance researchers at MIT have advanced strategies for detecting and mitigating gender and racial biases in natural language processing models. Stanford Universitys Institute for Human Centered Artificial Intelligence (HAI) is carrying out interdisciplinary studies on societal effect of AI which include troubles of fairness and bias.
Industry Initiatives
Many tech companies within USA are taking proactive steps to cope with bias in their AI systems. Google as an example has hooked up an Addressing Bias and Fairness in AI ethics board and posted principles for accountable AI improvement. IBM has released an AI Fairness 360 toolkit an open source library to assist hit upon and mitigate bias in device learning fashions.
Microsoft has developed recommendations for responsible AI and gear like Fairlearn which enables builders improve equity in their AI systems. Similarly Amazon has delivered SageMaker Clarify characteristic in its cloud platform that facilitates discover bias in gadget studying fashions.
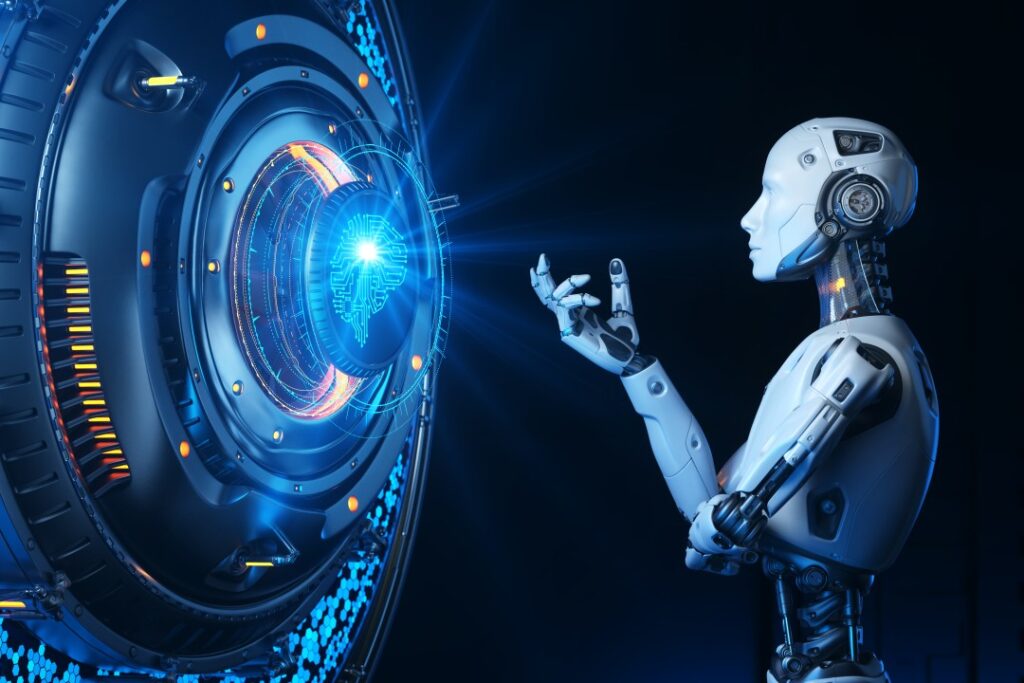
Government Action
The U.S. Authorities is likewise taking steps to address AI bias. In 2019 Senators Cory Booker and Ron Wyden brought Algorithmic Accountability Act which would require agencies to evaluate their AI structures for risks and biases. While this invoice has now not yet passed it reflects growing attention of need for regulation in this region.
The National Institute of Standards and Technology (NIST) has been operating on developing standards for AI such as guidelines for checking out AI structures for bias. Equal Employment Opportunity Commission (EEOC) has additionally all started to cope with implications of AI in hiring and employment choices.
Civil Society Organizations
Numerous non profit businesses and advocacy organizations inside USA are running to elevate attention about AI bias and push for greater equitable AI structures. AI Now Institute as an example conducts research and coverage advocacy targeted on social implications of AI. Algorithmic Justice League founded through Joy Buolamwini works to raise attention about influences of AI and develops practices for responsibility in AI.
Strategies for Mitigating AI Bias
Addressing bias in AI is complicated challenge that requires multifaceted technique. Here are some key strategies being employed and evolved in United States:
Diverse and Representative Data
One of fundamental ways to reduce bias in AI systems is to ensure that records used to teach these systems is diverse and representative. This entails no longer handiest amassing records from huge variety of resources but additionally carefully auditing this statistics for potential biases.
Some businesses are growing artificial datasets which can be designed to be more representative and balanced. Others are exploring techniques like facts augmentation to increase diversity of their training data.
Algorithmic Fairness
Researchers and builders are operating on creating algorithms which can be inherently more honest. This consists of growing new machine gaining knowledge of strategies which can optimize for each accuracy and fairness in addition to creating models which could detect and mitigate bias throughout training manner.
Techniques like adverse debiasing wherein model is skilled to are expecting target variable while simultaneously lowering its capacity to predict covered attributes are being explored as capability solutions.
Transparent and Explainable AI
Increasing transparency and explainability of AI systems is vital for detecting and addressing bias. This involves growing AI fashions that can provide clean factors for their choices making it easier to pick out whilst and way bias is influencing those choices.
Techniques like LIME (Local Interpretable Model agnostic Explanations) and SHAP (SHapley Additive exPlanations) are getting used to make black field AI models extra interpretable.
Regular Auditing and Monitoring
Implementing ordinary audits of AI systems for bias is crucial. This entails now not handiest trying out systems before deployment but also continuously tracking them in actual world applications to hit upon any rising biases.
Some corporations are growing automated gear for AI auditing while others are organising human in loop strategies to regularly assessment AI decisions for ability biases.
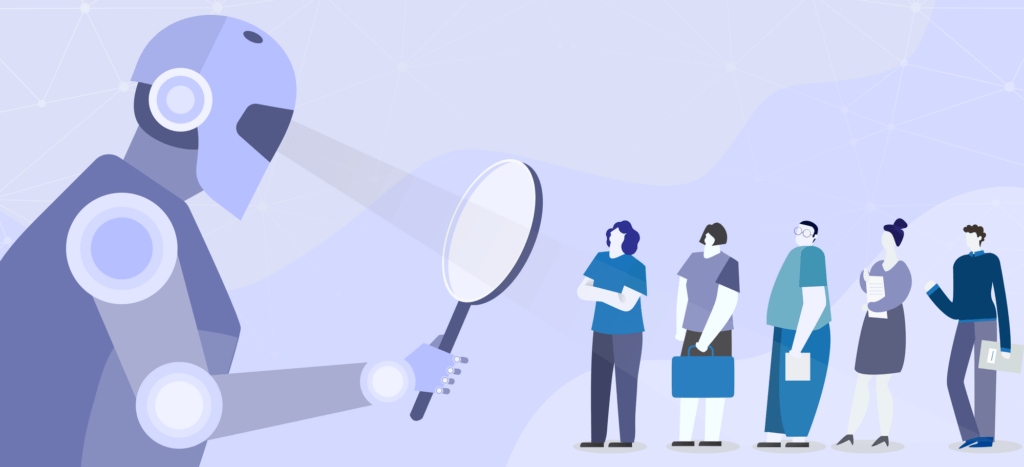
Diverse Development Teams
Increasing range in AI development teams is vital for bringing much broader range of views to design and implementation of AI systems. This entails no longer handiest hiring more numerous teams but additionally growing inclusive environments wherein all team contributors sense empowered to voice concerns about ability biases.
Ethical Guidelines and Governance
Many businesses are developing ethical recommendations for AI development and deployment. These guidelines frequently include concepts associated with equity and non discrimination in addition to procedures for ethical evaluate of AI tasks.
Some agencies also are organising AI ethics boards or committees to provide oversight and guidance on AI improvement and use.
Challenges in Addressing AI Bias
While good sized progress is being made in addressing AI bias inside USA numerous challenges stay:
Defining Fairness
One of fundamental demanding situations in addressing AI bias is problem in defining what constitutes “fairness” in distinctive contexts. There are multiple now and again conflicting definitions of algorithmic equity & selecting suitable definition can rely on specific application and societal context.
Balancing Fairness and Accuracy
In few instances efforts to make AI systems greater honest can come on price of decreased accuracy. Striking right balance between equity and standard system overall performance is an ongoing venture for AI builders.
Lack of Regulation
The lack of complete regulation around AI bias in USA poses challenges for making sure constant and effective approaches to addressing this problem. While few sectors (like finance and healthcare) have current regulations that may be carried out to AI many regions lack clean guidelines.
Proprietary Algorithms
Many AI structures utilized in crucial choice making tactics are proprietary making it difficult for outside researchers or auditors to take look at them for bias. This loss of transparency can avert efforts to hit upon and deal with bias.
Intersectional Bias
Addressing intersectional bias wherein couple of sorts of discrimination intersect is mainly tough. Most contemporary processes to mitigating AI bias focus on single attributes (like race or gender) however may also fail to capture more complex kinds of bias.
The Future of Fair AI inside USA
As USA maintains to grapple with challenges of bias in AI numerous trends are probable to form destiny panorama:
Regulatory Developments
Its possibly that well see accelerated regulation round AI bias within coming years. This could encompass obligatory impact checks for excessive hazard AI systems necessities for everyday auditing & standards for AI transparency and explainability.
Advances in Fair AI Techniques
Ongoing research is in all likelihood to yield new techniques for developing fairer AI systems. This ought to consist of more sophisticated processes to debiasing facts and algorithms in addition to new strategies for measuring and quantifying equity in AI structures.
Increased Public Awareness
As AI turns into more pervasive in regular existence public attention and challenge approximately AI bias are possibly to develop. This ought to lead to multiplied pressure on organizations and governments to deal with those issues.
Integration of Ethical AI in Education
Were possibly to see extra emphasis on ethics and fairness in AI training and schooling programs. This may want to assist ensure that following technology of AI builders is better equipped to address these challenges.
Cross Sector Collaboration
Addressing AI bias will probably require accelerated collaboration between academia enterprise authorities & civil society. We may see emergence of latest partnerships and tasks aimed at tackling those problems together.
Addressing bias and ensuring fairness in AI structures is one of maximum pressing ethical challenges dealing with america because it maintains to guide in AI innovation and deployment. affects of biased AI are some distance achieving referring to essential problems of equality justice & possibility in American society.
While good sized demanding situations continue to be developing awareness of these problems and concerted efforts throughout various sectors to deal with them are encouraging signs and symptoms. From modern day research in universities to proactive tasks via tech agencies from proposed rules to grassroots advocacy us is mobilizing on multiple fronts to address AI bias.
The path to honestly truthful and impartial AI is probably to be long and complex requiring ongoing vigilance innovation & collaboration. It will demand no longer handiest technical answers but also deep engagement with social cultural & historical contexts that provide upward thrust to bias inside first location.
As USA maintains to navigate those demanding situations choices made and procedures advanced may have profound implications now not only for American society but for worldwide development and deployment of AI.
By striving to create AI systems which can be fair and equitable USA has possibility to set powerful instance for rest of sector demonstrating how technological innovation may be harnessed to promote justice and equality.
The adventure in direction of honest AI is greater than technical project; its mirrored image of Americas ongoing battle to stay up to its ideals of equality and justice for all. As we circulate ahead addressing bias in AI may be crucial now not only for ethical improvement of generation however for very material of American society itself.